Artificial intelligence (AI) has grown rapidly, resulting in many classifications based on its functionalities. Two notable types of AI, generative AI and predictive AI, have received substantial interest in a variety of areas, including healthcare, finance, marketing, and entertainment. While both play important roles in automating jobs and improving human capacities, they use different mechanisms, use cases, and methodologies.
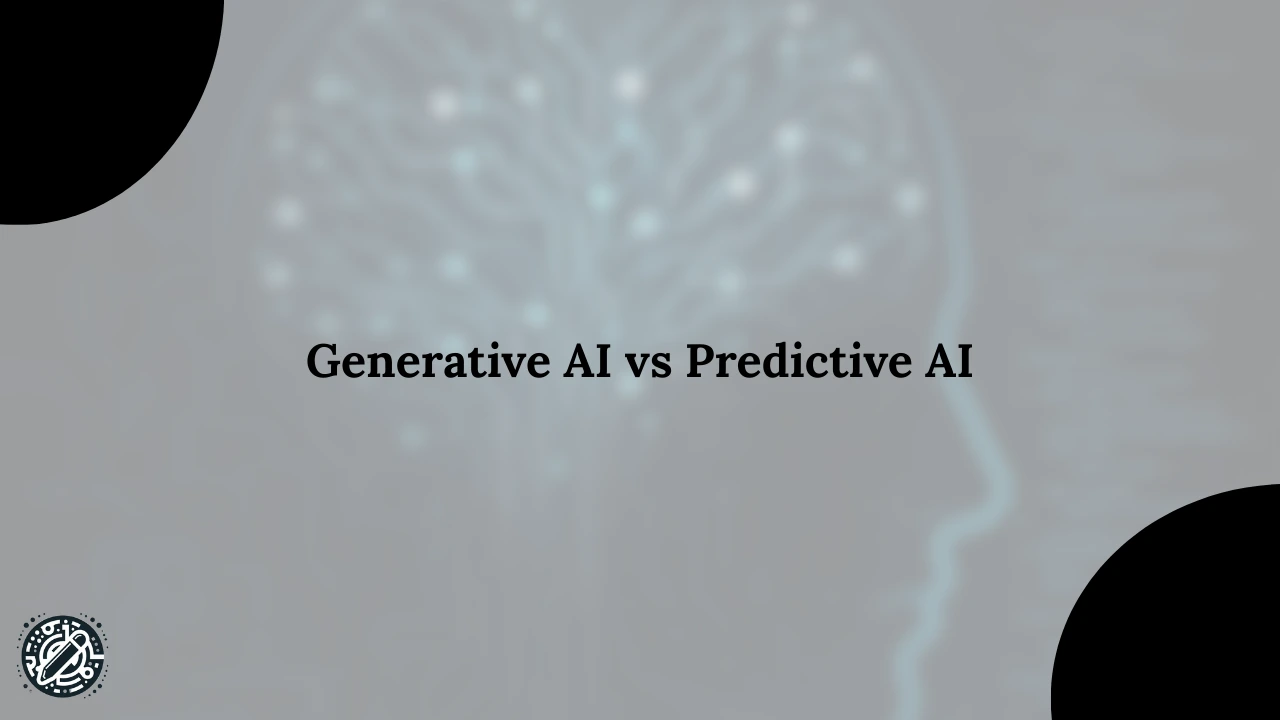
Table of Contents
This essay delves into the fundamental differences between Generative AI and Predictive AI, as well as its applications, underlying technologies, and future consequences.
Generative AI: Definition and Meaning
Generative AI is a type of artificial intelligence that creates new material rather than simply analyzing or categorizing current data. It learns from large datasets and produces unique results that resemble real-world patterns.
This technology enables AI chatbots, digital art, deepfake movies, and even AI-powered narrative, making it an essential tool for automation, creativity, and innovation across industries.
How Generative AI works?
Generative AI works by extracting patterns from vast datasets and applying powerful deep learning algorithms to generate fresh, realistic results. Three basic structures underpin generative AI:
Firstly, Generative Adversarial Networks (GANs) consist two competing neural networks. First one is generator that creates data, and a second one is discriminator that process evaluation of its authenticity. Generative AI models require extensive data sets for generating entirely new data. The data can be in the form of text, images and audio.
Secondly, Variational Autoencoders (VAEs) encodes input data into a compressed latent space. It undergoes training using machine learning algorithm. The AI starts recognizing patterns, relationships, and structures within the patterns. In text, it can be in the form of grammar rules and contextual meaning. In images, it can be in the form of recognizing shapes, texture, lighting, and depth.
Thirdly, after understanding the patterns and data, it starts making educated guesses about what should come next in a sequence. In text-based models like Chat GPT, it predicts the most probable next word in a sequence. Image generation models like DALL-E estimate the appropriate colors, textures or objects that fit into a generated image.
Lastly, Generative AI begins creating entirely original content by applying its learned knowledge. Remember, there is a saying that AI copies the information available inside the Pages and articles available on Search Engine Result Pages (SERPS). However, it is entirely incorrect statement. AI does not simply copy the existing data but recombines elements in new and innovative way.
Applications of Generative AI
Generative AI can be in various fields and industries. Generative AI provides creative, efficient, and innovative solutions.
It can be used in creating new content and provide ideas for marketing and advertising. AI-powered chatbots create high-quality data that can be used to modify blogposts, essays, and reports. AI generates personalized marketing copy, email campaigns, and product descriptions.
AI can also be used for AI art and design, deepfake technology, and 3D modeling and animation. Tools like DALL-E and MidJourney generate entirely new images from textual descriptions. In deepfake technology, AI alters videos to replace faces or create realistic avatars.
Tools like Google’s DeepComposer generate new melodies and harmonies. AI-driven voice models replicate human-like speech for virtual assistants and audiobook narration.
Strength and Limitations of Generative AI
The following are the strength of generative ai that provoke us for using Generative AI models:
- It enables creativity by generating unique and complex content. It can generate art, music, literature and even 3D designs. This allows artists, musicians, and designers to experiment with new styles.
- This automates content creation in industries like media and marketing. Content creators use AI tools like Jasper and copy.ai to generate blog posts, email campaigns, and ad copies. This significantly reduces the time and effort required for content production.
- This also enhances human productivity with AI-assisted designs and writings. In the realm of software development, tools like GitHub Copilot helps programmers by suggesting code snippets, improving efficiency and reduce errors.
The following are the limitations of Generative AI:
- There is also a possibility that it may produce inaccurate or biased content. Since AI models learn form existing data, it sometime leads to factually incorrect, misleading or biased outputs. Humans must verify the data before implanting on their personal spaces.
- It requires large datasets and computing power. This makes generative ai models expensive to develop and maintain.
- There are ethical concerns over deepfake generation and misinformation. By creating fake videos and images, it has become easy to raise questions on someone’s personality or company’s reputation. It can also be used for misinformation, fraud, and identity.
- Despite their advancement, there is limited control over output accuracy and coherence. Ensuring that AI remains aligned with user intent and factual correctness remain an ongoing challenge.
Predictive AI: Definition and Meaning
Predictive AI is a type of artificial intelligence that focuses on analyzing patterns in data to forecast future events or behaviors. Generative AI focuses predominantly on creation of new content, on the other hand, predictive AI is primarily used for decision making, trend analysis, and risk assessment across various industries.
Predictive AI is contributing heavily in the field of finance, healthcare, marketing, and cybersecurity. In the later part of this article, we will cover how it works, its applications, advantages, challenges, and future trends.
What is Predictive AI?
Predictive AI refers to artificial intelligence models that use historical data, statistical algorithms, and machine learning techniques to make informed predictions about future outcomes. Predictive AI analyzes patterns, trends, and correlations to anticipate what will happen next.
How Predictive AI works?
Predictive AI follows a structure process that involves data collection, preprocessing, model training, and prediction generation.
The following are several machine learning models that power predictive AI:
- Regression Models are used to establish relationship between variable and predict continuous outcomes. Well-known examples are Linear regression (used in real estate pricing) and logistic regression (used in fraud detection).
- Another model is Decision Trees and Random forests. Decision tree split data into branches based on conditions, while Random Forests combine multiple decision trees for higher accuracy. For example, credit score assessment for loan approvals.
- Artificial Neural Networks (ANN) mimic the human brain’s functioning to detect pattens, These Neural Networks and Deep Learning models are used in speech recognition, image classification, and disease diagnosis.
- Time Series Analysis is used for forecasting trends over time, such as stock market predictions or weather forecasting.
- Natural Language Processing based predictive models analyze customer sentiment in reviews, social media and survey. For example, AI predicting the success of a movie based on public sentiment.
Training Process of Predictive AI
It follows the following process:
- Historical data is gathered from multiple sources.
- Data preprocessing
- Identification of key variables that influence predictions
- AI learns patterns using machine learning algorithms
- Validation and testing
- Lastly, the trained model generates forecasts.
Applications of Predictive AI
Although, predictive AI has transformed numerous industries, but it is commonly used in healthcare, and finance and banking.
Artificial Intelligence is used to make stock market predictions. AI analyzes buying and selling trends to forecast stock prices and investment opportunities.
In banking, AI models identify unusual transaction patterns, reducing financial fraud risks.
Similarly, in healthcare sector, AI can help doctors detect diseases early by analyzing patient records and medical history. Predictive models recommend personalized treatments based on genetic data.
Advantages and Challenges
Predictive AI provides data driven insights. Along with that it optimizes resource allocation, reducing waste and operational costs. It automates repetitive tasks and identifies potential risks.
Despite its advantages, predictive AI faces several limitations. Incomplete or biased data can lead to incorrect forecasts. Its system analyzes vast amount of personal data which raises privacy concerns.
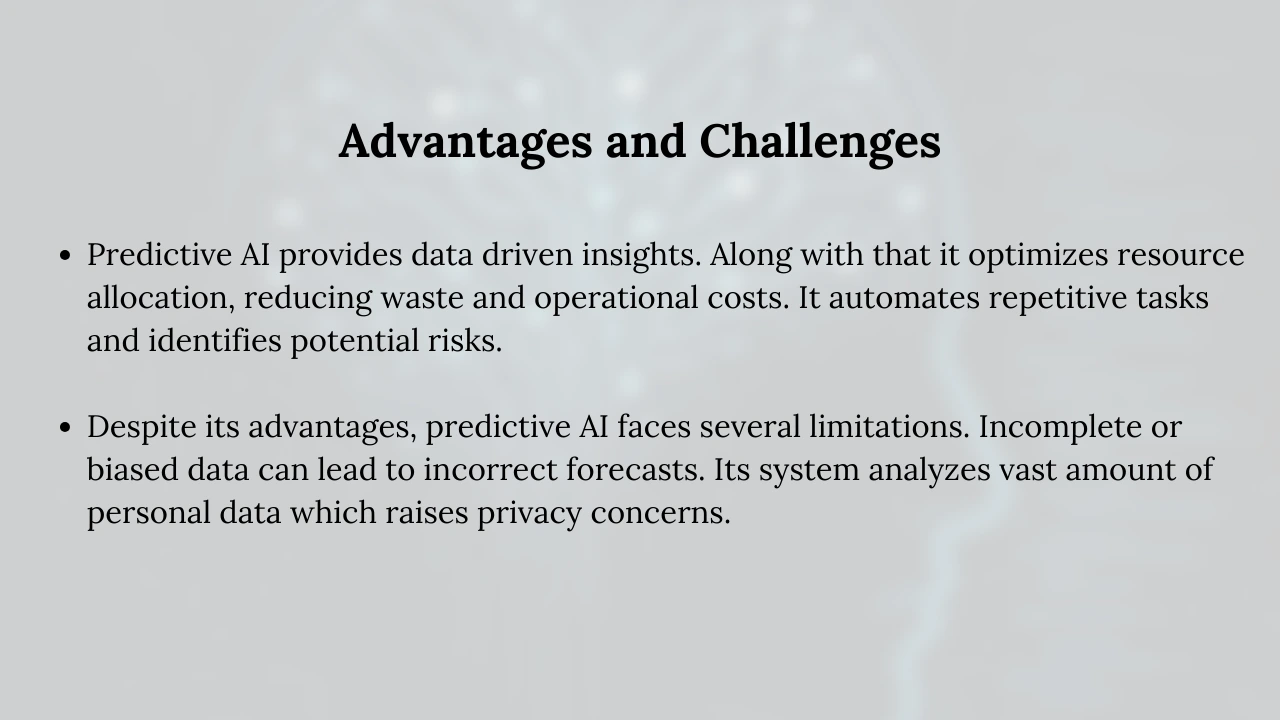
Final Thoughts
Generative AI excels at creating new and original content by learning patterns from data, on the other hand, predictive AI focuses on forecasting future events based on historical trends. Together, these two products of AI to enhance creativity, improve decision-making, and drive innovation across industries. However, both fields come with challenges around bias, accuracy, and ethical use.
FAQs
What are common applications of Predictive AI?
Predictive AI is widely used in finance for fraud detection, in healthcare for disease prediction, in marketing for customer behavior forecasting, and in manufacturing for predictive maintenance.
What are the limitations of Generative AI?
Generative AI may produce biased, inaccurate, or unethical content, requires large datasets and heavy computing power, and sometimes lacks control over output precision.
What are the challenges of using Predictive AI?
Predictive AI depends on the quality and quantity of historical data, may suffer from bias, and raises privacy and ethical concerns if not properly managed.
Are there ethical concerns with both Generative and Predictive AI?
Yes, Generative AI can lead to misinformation (e.g., deepfakes), while Predictive AI can result in biased or unfair decision-making if trained on flawed data.